All published articles of this journal are available on ScienceDirect.
Biochemical Variables are Predictive for Patient Survival after Surgery for Skeletal Metastasis. A Prediction Model Development and External Validation Study
Abstract
Background:
Predicting survival for patients with metastatic bone disease in the extremities (MBDex) is important for ensuring the implant will outlive the patient. Hitherto, prediction models for these patients have been constructed using subjective assessments, mostly lacking biochemical variables.
Objectives:
To develop a prediction model for survival after surgery due to MBDex using biochemical variables and externally validate the model.
Methods:
We created Bayesian Belief Network models to estimate likelihood of survival 1, 3, 6, and 12 months after surgery using 140 patients. We validated the models using the data of 130 other patients and calculated the area under the Receiver Operator Characteristic curve (ROC). Variables included: hemoglobin, neutrophil-count, C-reactive protein, alkaline phosphatase, primary cancer, Karnofsky-score, ASA-score, visceral metastases, bone metastases, days from diagnose of primary cancer to index surgery for MBDex, ischemic heart disease, diabetes, fracture/impending-fracture and age.
Results:
Survival probabilities were influenced by all biochemical variables. Validation showed ROC for the 1, 3, 6, and 12-months model: 68% (C.I.: 55%-80%), 69% (C.I.: 60%-78%), 81% (C.I.: 74%-87%) and 84% (C.I.: 77%-90%).
Conclusion:
Biochemical markers can be incorporated into a prediction model for survival in patients having surgery for MBDex allowing surgeons to offer more objective and individualized treatment options.
1. INTRODUCTION
Successful treatment of metastatic bone disease in the extremities (MBDex) requires a multidisciplinary approach. Most lesions may be treated in a non-surgical manner by incorporating radiation therapy, bisphosphonates, and pain management [1, 2]. However, in case of a pathological fracture or intractable pain, surgery is often necessary [3]. Various surgical implants such as internal fixation devices or joint replacement prostheses can be used, but each has different indications, complication profiles and rehabilitation requirements. To determine which patients may benefit from surgery, and, to determine whether a more durable implant may be necessary, surgeons need a tool that can help predict each patient’s residual life expectancy.
The definitive surgical strategy is often considered within a multidisciplinary environment. Factors such as anatomical location of the metastatic lesion, information regarding the primary cancer causing the lesion, and the general health status of the patient influence surgical decision-making. However, the patient’s life expectancy is central to choosing an effective surgical strategy.
Unfortunately, survival estimates made by health care professionals are inconsistent, at best. Most providers tend to overestimate survival, which can lead to overtreatment, however, undertreatment is also a problem in these patients who are terminal, but not necessarily terminally ill. A prognostic tool containing objective variables is therefore necessary [4-6].
Several risk factors for survival in patients having surgery due to MBDex have been identified [1, 2, 7-12] of what many variables are observer depended (e.g. performance status or surgeons estimate of residual life expectancy). Objective factors such as biochemical variables have been investigated in patients with metastatic bone disease, but usually are employed as an “independent” predictor for survival, and as yet, have not been part of multivariate models designed to estimate survival. Multivariate models containing hemoglobin, and absolute lymphocyte count—in addition to a combination of objective and subjective information-are used to estimate the duration of survival and have been externally validated [13, 14]. However, C-reactive protein [15, 16], alkaline phosphatase [17-21] and neutrophil count [21-24] previously shown to be independent risk factors for survival have not yet been investigated in the context of a multivariate model for survival prediction in MBDex patients.
With this in mind, we aim to investigate if a multivariate prediction model for survival after surgery due to MBDex can be built using biochemical variables and furthermore; investigate how such model perform in an external validation.
2. MATERIAL AND METHODS
2.1. Study Design and Setting
This study obtained ethical approval by the Danish Health Authorities (ID-no: 3.3013-880/1) and Danish Data Protection Agency (ID-no: 30-1222). Patients were identified from the COpenhagen BOne Metastases Database (COBOM database [25]), which contains retrospectively collected clinical data from January 1st 2003 to 31st December 2013 of patients treated in our center for MBDex with joint replacement surgery or a diaphyseal spacer. Our center is one of two tertiary referral centers for orthopedic oncology in Denmark and therefore the COBOM database can be considered to be representative for a population based cohort of patients having highly specialized treatment for MBDex. As no consecutive list has been kept for patients being treated by internal fixation methods, these patients are excluded from the COBOM database to eliminate selection bias of long term survivors and as treatment philosophy in our center is to prefer bone resection and reconstruction, this number is expected to be low.
2.2. Study Population
All patients who contributed with data for the current study underwent surgery for symptomatic MBDex. We collected information that would have been available prior to surgery from the electronic medical record. Data from patients who underwent subsequent surgeries during the inclusion period was only included with the first operation in the study period, thus not to violate the assumption of independence [26]. Inclusion criteria were: surgery due to histology-proven MDB, with joint replacement surgery (with or without bone resection) or bone resection and replacement with diaphyseal spacer of a long bone. Exclusion criteria were: surgery in the spine, revision surgery for failed implants, other types of surgery such as intramedullary nailing or plate fixation, age under 18 years, and the absence of malignant cells present in resection material. Patients with hematological disease of bone were included in this study, hence the same surgical treatment approach is used as with metastases caused by solid cancers. Decision-making for surgery or palliative treatment was based in case-by-case multidisciplinary team evaluation and patient involvement.
We divided the dataset into two groups, 1 for model development, and 1 for validation based on when electronical patient files was introduced. The first (training cohort), that consisted of all patients having joint replacement surgery or a diaphyseal spacer from January 1st 2009 to December 31th 2013 [27], was used to build the prediction models (n = 140). The second (validation cohort), included patients treated from January 1st 2003 to December 31th 2008 (n = 130), was used to test each model’s performance. Each model was trained to estimate the likelihood of survival (yes/no) at 1, 3, 6 and 12- months after surgery. Due to the Danish Civil Registration System no patients were lost to follow-up [28].
2.3. Prognostic Variables and Dichotomization
Explanatory variables for survival were chosen upon review of the literature and included: primary cancer, grouped according to Forsberg et al. [29], in brief slow growing cancer with long expected survival (breast, prostate, renal cell, thyroid cancer, myeloma and lymphoma), moderate growing cancers (sarcoma and other carcinoma) and fast growing cancers (lung, gastric, hepatocellular and unknown origin). Other included variables were: age at surgery, days from primary cancer diagnosis to index surgery, presence of visceral metastases, solitary or multiple bone metastases, ischemic heart disease, diabetes, Karnofsky performance score (KPS) [30], The American Society of Anesthesiologists Physical Status classification system (ASA-score) [31], bone fracture or impending fracture, and preoperative biochemical variables (hemoglobin level, neutrophil count, C-reactive protein, alkaline phosphatase). Visceral metastases were evaluated by preoperative CT scans and considered positive, if any dissemination to soft tissue or lymph nodes were present on scans performed within 3 months prior to surgery. If no scans were performed, this variable was considered missing.
Biochemical variables older than 7 days prior to surgery were also considered as missing variables.
Gender was excluded from the analysis based on its close relation to primary cancer type.
We categorized by reference interval for normal range (neutrophil count) or by interval described in the literature for survival in patients having surgery for MBDex (KPS). If no consistent scientific evidence for a reference interval regarding prediction of survival in patients having surgery due to MBDex could be found in the literature, we then categorized by the median for the combined cohort. This dichotomizing strategy was chosen to minimize confounding of the data and loss of power.
We categorized KPS ≥ 70 or < 70 (able to work or Eastern Cooperative Oncology Group performance score [32] <1 or >2), ASA score ≤2 or ≥3, hemoglobin ≥ 8 mmol/L (median) or < 8 mmol/L, neutrophil count ≥ 7.9 x109/L (reference interval) or < 7.9 x109/L, C-reactive protein ≥ 30 mg/L (median) or < 30 mg/ml, alkaline phosphatase ≥ 130 U/L (median) or < 130 U/L, age ≥ 65 years of age [8] or < 65 years of age, days from diagnose to surgery ≥ 757 (median).
2.4. Development of Prediction Model
Each of the above variables was considered as candidate features for inclusion into the model. We produced a prediction model for survival 1, 3, 6 and 12- months after surgery using a Bayesian Belief Network (BBN) [33] with patients from the training cohort. The purpose of the 1-month model is to assist the surgeon in decision-making between pure palliative treatment or surgical treatment. The 3-month model could be used to guide the surgeon between how durable an implant should be in case of fracture or palliative treatment of an impending fracture. The 6 and 12-month models were produced to assist the surgeon in choosing a somewhat more durable/invasive implant to ensure on surgical implant that will outlive the patient.
We used FasterAnalytics™ v7.0 [34], which employs an unsupervised machine-learning algorithm to calculate joint probability distributions-how and under what conditions one variable may be represented in terms of other variables-without defining an a priori hypothesis or designating an outcome variable. All candidate features are considered during this feature selection process. One of the strengths of using a Bayesian model is its ability to function when some input data is missing. For the end user, imputation of missing values is unnecessary, which makes it well-suited for the clinical setting where clinicians are sometimes faced with making decisions with incomplete information.
2.5. Statistical Analysis
We addressed model performance according to guidelines presented by Steyerberg et al. [35]. Discriminative ability was described using Receiver Operating Characteristic (ROC) and measurement of Area Under the Curve (AUC) by producing predictions of survival for each patient in the validation cohort. Decision Curve Analysis (DCA) [29, 36] was performed to determine what thresholds in the data may be useful for clinical decisions. DCA is a method for evaluating prediction models in the absence of specific outcome metrics, overcoming a limitation of traditional decision analysis methods. DCA is utilized to compare prediction models and, importantly, determine whether models are indeed suitable for clinical use.
Distributions of variables between the two cohorts were tested for equality with non-parametric tests. Continuous data with Mann-Whitney-Wilcoxon test and categorical data with chi-squared (CHI2) test. We used Kaplan-Meier estimate to compare overall survival between groups. Overall survival for these two groups has previously been described by Hovgaard et al. [25]
3. RESULTS
3.1. Comparison of Training and Validation Cohorts
All records contained follow-up sufficient to establish survival at 12 months post-surgery.
Patient demographics can be addressed in Tables 1 and 2. The cohorts contain no differences in age, primary cancer, fracture, visceral metastasis, solitary metastasis, ASA score or KPS between the test and validation cohort [25]. However, days from the diagnosis of cancer to surgery for MBDex did differ between the two cohorts (p < 0.001) with a shorter period for the validation cohort.
- | Training cohort (2009-2013) n=140 |
Test cohort (2003-2008) n = 130 |
- | - | |||
---|---|---|---|---|---|---|---|
Variable | - | - | Missing (%) |
- | Missing (%) |
Mann-Whitney-Wilcoxon test | Chi2 test |
Age at surgery (years) | Mean | 64.7 | 0 | 63.7 | 0 | 0.531 | N/A |
- | Median | 65.0 | - | 64.0 | - | - | - |
- | Range | 21 - 90 | - | 30 - 85 | - | - | - |
Hemoglobin | Mean | 7.1 | 0 | 7.4 | 1 | *0.007 | *0.030 |
(mmol/L) | Median | 7.1 | - | 7.5 | - | - | - |
- | Range | 4.8 - 9.8 | - | 5.0 - 9.7 | - | - | - |
C-reactive protein | Mean | 48.1 | - | 68.5 | 15 | *0.018 | *0.038 |
(mg/L) | Median | 21.0 | - | 37.0 | - | - | - |
- | Range | 1 - 329 | 4 | 0 - 266 | - | - | - |
Neutrophil count | Mean | 6.8 | 16 | 6.7 | 32 | 0.757 | 1 |
(x 10^9/L) | Median | 5.6 | - | 5.8 | - | - | - |
- | Range | 0.2 - 25.1 | - | 1.5 - 18.0 | - | - | - |
Alkaline phosphase | Mean | 182.5 | 16 | 289.6 | 9 | *0.006 | *0.031 |
(U/L) | Median | 122.0 | - | 158.0 | - | - | - |
- | Range | 39.0 - 796.0 | - | 49.0 - 2520.0 | - | - | - |
Days from diagnose to surgery |
Mean | 1468 | 0 | 1113 | 0 | *<0.001 | *0.004 |
- | - | - | - | - | - | - | |
(Days) | Median | 667.6 | - | 152.5 | - | - | - |
- | Range | 0 - 10610 | - | 0 -31180 | - | - | - |
Due to a high percent of missing values for diabetes and ischemic heart disease, we choose not to perform statistical test on these variables. Biochemical variables were differently distributed across the cohort for all parameters excluding neutrophil count (p = 0.757), (Tables 1 and 2).
- | - | Training cohort | Validation cohort | - | ||
---|---|---|---|---|---|---|
Variable | - | No. | Missing (%) | No. | Missing (%) | Chi2 test |
Fracture | - | - | - | - | - | - |
- | Yes | 104 | 0 | 94 | 0 | 0.819 |
- | No | 36 | - | 36 | - | - |
Cancer group | - | - | - | - | - | - |
- | Slow | 99 | 0 | 79 | 0 | 0.225 |
- | Moderate | 10 | - | 13 | - | - |
- | Fast | 31 | - | 38 | - | - |
Visceral metastases | - | - | - | - | - | - |
- | Yes | 47 | 0 | 58 | 8 | 0.083 |
- | No | 93 | - | 64 | - | - |
Skeletal metastases | - | - | - | - | - | - |
- | Solitary | 53 | 0 | 38 | 0 | 0.228 |
- | Multiple | 87 | - | 92 | - | - |
ASA group | - | - | - | - | - | - |
- | 1 + 2 | 63 | 7 | 74 | 2 | 0.147 |
- | 3 + 4 | 70 | - | 54 | - | - |
Karnofsky score | - | - | - | - | - | - |
- | >= 70 | 96 | 0 | 73 | 3 | 0.095 |
- | < 70 | 44 | - | 53 | - | - |
Ischemic heart disease | - | - | - | - | - | - |
- | Yes | 6 | 0 | 0 | 128 | N/A |
- | No | 134 | - | 2 | - | - |
Diabetes | - | - | - | - | - | - |
- | Yes | 19 | 0 | 0 | - | - |
- | No | 121 | - | 2 | 128 | N/A |
3.2. Bayesian Belief Network Structure
BBNs were produced for all four survival endpoints. The first-degree associates - those variables that were most influential on 1, 3, 6 and 12-months survival, included: 1 month: KPS, 3 months: KPS, visceral metastasis, alkaline phosphatase, neutrophil count, 6 months: KPS, alkaline phosphatase, neutrophil count, hemoglobin and 12 months: KPS, alkaline phosphatase, neutrophil count, hemoglobin, C-reactive protein and primary cancer group (Figs. 1-4). The number of skeletal metastases, diabetes, bone fracture and age did not add any prognostic information and were excluded as part of the machine learning process.
3.3. Model Performance
Validation showed that our models performed very strongly in predicting survival at 6 and 12 months AUC for the 6 and 12 months models were 81% (C.I.: 74% - 87%) and 84% (C.I.: 77% - 90%), respectively. Discriminatory ability was fair for the 1 and 3-month models: 68% (C.I.: 55% - 80%), and 69% (C.I.: 60% - 78%), respectively.
On DCA, all four models are suitable for clinical use, and demonstrate positive net benefit, rather than assuming all patients or no patients survive (Figs. 5-8) 1, 6, or 12 months, respectively. However, with the 3-month model, a surgeon is better off assuming all patients will survive 1 months if his or her threshold probability for treatment is above 0.58. Surgeons typically have higher thresholds for treating sicker patients, and lower thresholds for treating healthier patients. Likewise, DCA analysis shows that clinical use of the 1-month model is suitable for treatments thresholds exceeding 0.72.
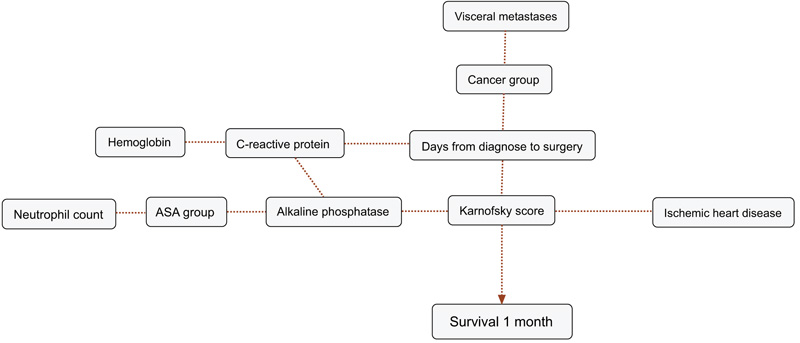
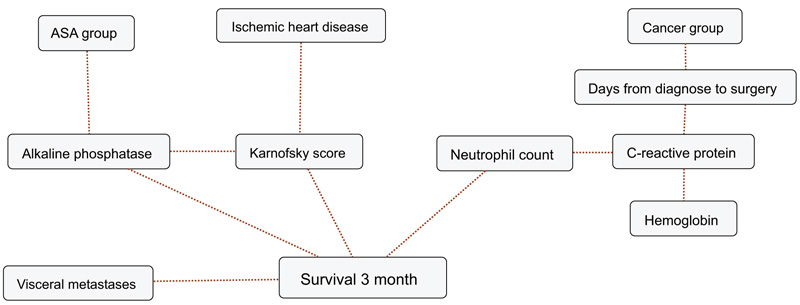
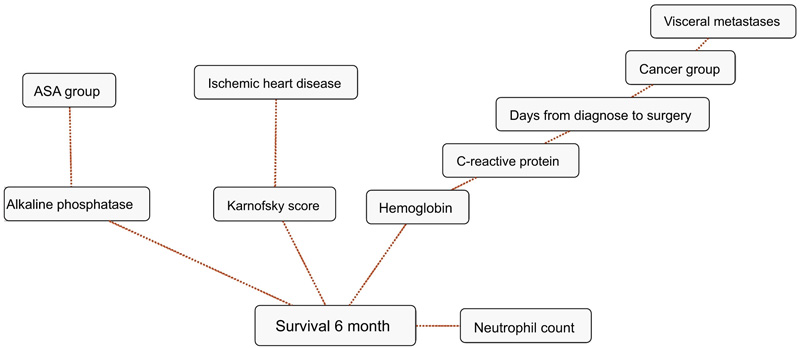
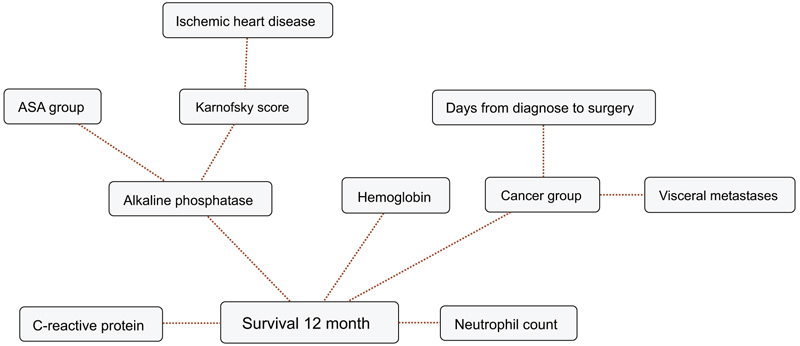
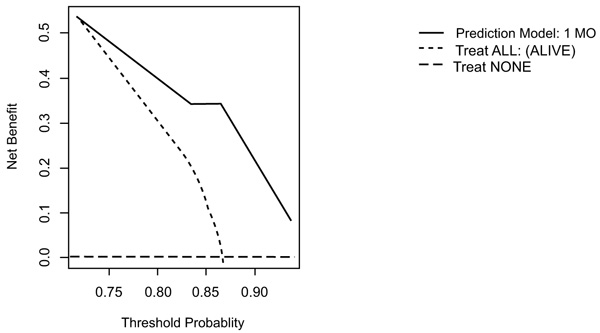
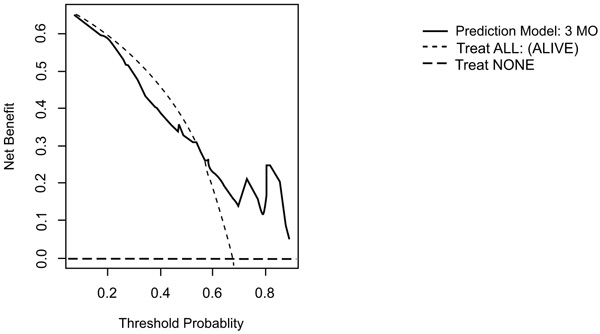
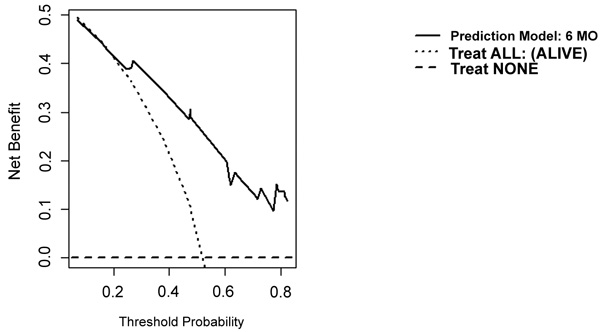
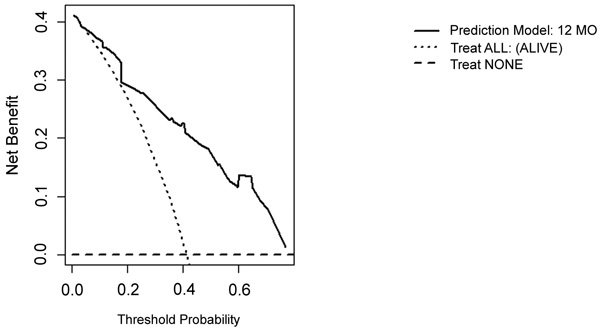
4. DISCUSSION
The treatment of patients with MBDex is dependent, in large part, on each patient’s estimated survival. Herein, we demonstrate that biochemical variables (C-reactive protein, hemoglobin, neutrophil count and alkaline phosphatase), can be included into a multivariate model to estimate the likelihood of survival after surgery for MBDex. In addition, we provide validation data, and decision analysis, which indicates the models may be used in a clinical setting.
4.1. Limitations
Our study is limited by several factors and our results should be interpreted with these in mind; Firstly, data arose from a single institution although from a prospective maintained database the data is considered retrospective. Secondly our institution is a tertiary referral center and hereby our population does not truly reflex a population based cohort of patients having surgery for MBDex. This may result in overfitting of the analysis in a BBN and result in overly optimistic results in DCA. As illustrated by the validation of PATHFx [37, 13, 14], it is a problem to find a cohort of patients having surgical treatment for MBDex with limited missing data. As such, our priority was to use a validation cohort with a minimum of missing data instead of an independent cohort with large amounts of missing data.
One may ask why the orthopedic community needs additional prediction models when several exist? First, the methods we use to estimate survival must be able to accommodate new data, as treatment philosophies change, and more effective means of treating MBDex become available. By including biochemical markers, we show that each (hemoglobin level, neutrophil count, C-reactive protein, alkaline phosphatase) has predictive value, and may be used as candidate features for future generations of models. In addition, scoring systems developed from data collected of patients having nonsurgical treatment may impart a time-dependent bias [38], mainly because this treatment generally occurs prior to the time when the lesion(s) require surgical intervention. Using models developed in this fashion [1, 2, 39], we would tend to overestimate survival and risk over-treating the patients. By only including data available at time of surgery, we thereby eliminate this bias.
It is important that prognostic models are developed within the context of a defined medical problem, in a specific patient population. Balachandran et al. [40] underline the importance of correct construction, interpretation and application of medical prediction models since they find these incompletely understood by the medical community. By focusing on patients requiring orthopedic surgical intervention for MBDex, we attempt to overcome this problem by choosing a cohort that we find is representative of the population.
It is possible that other techniques than BNN may be used to estimate survival. Janssen et al. [8] evaluated three different methods for development of a prediction model for survival after surgery due to MBDex. In conclusion, they found that the performance of a boosting algorithm and a nomogram had equal performance. The authors advocated the use of a nomogram over the boosting algorithm as they find it more easily used in clinical settings. However, the use of a nomogram forces the clinician to have complete input data, which is not always available in the clinical setting, which may impart delays in treatment, or limit its use. To mitigate this, Forsberg et al. [29] made a series of Bayesian models publicly available on www.pathfx.org and made it easily usable in clinical setting.
The choice of a BBN for construction of the prediction model enables us to deal with missing data for the individual patient without excluding them from analysis. Missing data is a problem in clinical settings specifically in the case of a fracture where intractable pain of the lesion causes the need for urgent decision-making and leaves little time to further elucidation if not absolutely indicated.
In the present study, we included biochemical markers that are commonly used in the perioperative setting, but had not been used in other prediction for survival models. Each has demonstrable prognostic value in estimating survival in patients with advanced cancer [12, 2, 23, 22, 24, 17-21, 41, 15, 11, 7]. In doing so, we produced models with high discriminatory capacities in an independent cohort, similar to the internal validation statistics reported by Janssen et al. [8] as well as the external validation of PATHFX by Forsberg et al. [18], Piciolli et al. [13] and Ogura et al. [37]. This is an important observation, since models exhibit a decrease in discriminative ability when presented with unknown records during external validation. As such, models that have not undergone external validation in an intended patient population are not recommended for clinical use.
To our knowledge, no other models other than Janssen and PATHFx for prediction of survival in patients having surgery for MBDex have been externally validated. None of the mentioned models includes C-reactive protein, which has shown to be a strong predictor for survival in patients with cancer [16, 15] nor alkaline phosphatase [17, 19-21]. No studies have addressed the connection between survival in patients having surgery due to MBDex and neutrophil count, however, Forsberg et al. [42] included absolute lymphocyte count (off which neutrophil count is a subset) in the PATHFX model. Literature underline that neutrophil count is associated with survival in cancer patients with metastatic bone disease [21-24] and this is coherent with findings in this study. Biochemical variables are objective variables opposed to KPS, ASA-score etc. A non-objective variable is dependent on the observer’s qualification and can therefore bias the outcome of a prediction model for the individual patient. By including more objective variables, such as biochemical variables, we believe that our model becomes more robust and less observer dependent and thus minimizing observation bias in our model.
The clinical rationale for development of prediction models for survival in patients having surgery for MBDex is to produce a tool that can guide the surgeon into choosing the right treatment for the individual patient, so the patient only need one operation and can avoid revision surgery. We choose to develop the 1-month model to assist the surgeon or the medical oncologist in choosing between surgery or palliative treatment. Prediction horizons of 3-, 6- and 12 months was produced to assist the surgeon in choosing between a more or less durable implant (internal fixation versus joint replacement), as it has been shown that the use of intern fixation in long term survivors will result in revision due to implant failure [43, 44] as one cannot expect a metastatic lesion to heal, not even under radiation therapy [45]. Even though a recent review [46] of musculoskeletal tumor surgeons has shown that survival predictions of 6 months after surgery is of interest for choosing between various surgical treatment modalities for the proximal femur, the authors feel that the clinical reality is not always as straight forward, and the need for prediction of shorter (3 months) or longer (twelve months) survival is of interest in case of pending surgical treatment for metastatic lesions also in other anatomical regions than the proximal femur and was thus included into our prediction model.
We choose to evaluate the usefulness of the models by DCA. DCA is about evaluating the net benefit of using a model compared to assuming all patients either survive or dies at different thresholds for individual survival predicted by a given model. In clinical settings, a surgeon might require a high probability of survival before offering a costly procedure to a patient with a high risk of perioperative/postoperative complication. If this survival probability is lower than the threshold probability for the given model in a DCA analysis the surgeon is better off not using the model at all.
DCA analysis of the 1-month model suggests using the model rather than assuming a patient will survive/die if we demand a 72% or higher probability of surviving one month.
Interestingly the 3-month model yields negative net benefit at threshold below 0.52 compared to the clinician expecting all to survive and it is therefore not recommended to use the model at lower thresholds. Piccioli et al. [13] observed similar findings in DCA analysis of the PATHFX 3-month model although they found a threshold at 0.90, which is increased to our findings (0.52). Ogura et al. [37] observed something similar for their 1 month (0.80) and 3-month prediction model (0.60). This large difference of net benefit across threshold probabilities can be explained by our two cohorts being very homogeneous (most variables have the same distribution in the training and the validation cohorts and no significant difference in survival pattern was observed) in contrast to the training and various validation cohorts of the PATHFx.
The authors underline that the result of this study is only applicable for patients whom is designated to undergo surgery for MBDex and not for those where non-surgical interventions is the clinician first choice of treatment for a metastatic lesion, and a recently published study documented that one could expect approximately below 80% of the patients admitted to an orthopedic department suffering from MBDex will be treated surgically [47].
CONCLUSION
In conclusion, we generated prediction models designed to estimate the likelihood of survival at four useful time-points also including common biochemical markers together with primary cancer type, presence of visceral metastasis, KPS, ASA group, ischemic heart disease, days from diagnosis of cancer to surgery for MBDex; we ensured each model was suitable for clinical use using decision analysis. These models may now be used to assist the surgeon or the medical oncologist in their clinical decision making when treating MBDex.
LIST OF ABBREVIATIONS
ASA | = American Society of Anesthesiologists score |
AUC | = Areal Under the Curve |
BBN | = Bayesian Belief Network |
COBOM database | = COpenhagen BOne Metastasis Database |
DCA | = Decision Curve Analysis |
KPS | = Karnofsky Performance Status |
MBDex | = Metastatic Bone Disease in the extremities |
ROC | = Receiver Operating Curve |
ETHICS APPROVAL AND CONSENT TO PARTICIPATE
This work was evaluated and approved by the Danish Health Authorities (ID-no: 3.3013-880/1) and Danish Data Protection Agency (ID-no: 30-1222).
HUMAN AND ANIMAL RIGHTS
The reported experiments are in accordance with the ethical standards of the committee responsible for human experimentation (institutional and national), and with the Helsinki Declaration of 1975, as revised in 2013 (http://ethics.iit.edu/ecodes/node/3931)
CONSENT FOR PUBLICATION
Written informed consent was obtained from all participants.
CONSENT FOR PUBLICATION
MSS, KH: None.
MMP: Research support unrelated to this study from Zimmer-Biomet, Ethicon UK, and Bonesupport.
JAF: Zimmer-Biomet, research support as PI, unrelated to this project. Clementia Pharmaceuticals, Former paid consultant for protocol development, unrelated to this project.
ECS and SK: Employees of DecisionQ, Inc. Washington DC.
US Government Disclaimer:
The views expressed in this article are those of the authors and do not necessarily reflect the official policy or position of the Department of the Navy, the Department of Defense, nor the U.S. Government. Research activities leading to the development of this abstract were approved by the local Institutional Review Board (NMRC.2014.0009 and IRBNet 392350) in compliance with all applicable regulations governing the protection of human subjects. One of the authors (JAF) is a military service member. This work was supported/funded by work-unit number HU0001-14-1-0010 and was prepared as part of his official duties. Title 17 U.S.C. §105 provides that “Copyright protection under this title is not available for any work of the United States Government”. Title 17 U.S.C. §101 defines a U.S. government work as a work prepared by a military service member or employee of the U.S. Government as part of that person’s official duties. We certify that the document represents valid work; that if we used information derived from another source, we obtained all necessary approvals to use it and made appropriate acknowledgements in the document; and we take public responsibility for it.
ACKNOWLEDGEMENTS
Declared none.